In this article, we’ll discuss current best practices, tech trends, and the future of data analytics. Data analytics is the process of preparing, processing, mining, and interpreting data to extract valuable insights and empower informed decision-making in business.
Surviving in the market pushes many organizations to master data analytics trends as they promise higher efficiency and offer growth-hacking scenarios. This evokes vigorous interest in the value of data analytics as a prerequisite for optimization in enterprise management, identifying business opportunities, and driving organizational change in the right direction.
With the help of data analytics, business executives can achieve the following effects:
- Find data patterns and trends in the business metrics and outcomes (like seasonal fluctuations in sales) and make pattern-based predictions;
- Identify business bottlenecks and shape strategies to overcome them;
- Determine normal and superfluous levels for different operational metrics to formulate what’s good and what’s bad in more precise and reality-focused terms;
- Build complex correlative models that describe exact relationships between factors/causes and business outcomes;
- Define data abnormalities and phenomena in business operation results;
- Turn all findings and conclusions into efficient business management reactions, methods, and decisions.
Why is it necessary to embrace the modern trends in data analytics for business success? There are several reasons for popularizing data analytics:
- Informed decisions are confirmed to be more efficient than pure reliance on intuition or professional experience. This is because data analytics excludes different emotional factors and cognitive distortions specific to humans;
- The wide availability of powerful computers and mobile devices;
- A wide selection of data analytics applications and methods;
- The possibility for business data to be accumulated in enterprise systems;
- Decreased requirements for mathematical skills in users.
The last point is extremely important, as modern data analytics can run automatically or semi-automatically. Thanks to auto-generated analytical reports and takeaways delivered by modern software products, business managers and executives don’t need to possess advanced statistician skills to perform data research.
The latest techniques and trends in analytics include machine learning, artificial intelligence, big data, data governance, and data visualization. All these methods can be used either alone or in combination. Let’s learn more about each of them.
Can ML Technology Learn as Organic Brains Do?
The data analytics future can hardly be imagined without machine learning (ML.) This methodology of modern data science involves the use of intelligent algorithms and statistical matrix models empowering computer systems to “learn” and accumulate experience to improve their performance on a specific task or problem.
ML role in data analytics
The basic idea behind ML is to let machines acquire experience automatically instead of being explicitly programmed. ML replicates natural learning abilities specific to all living beings. This requires a machine “training” routine: the computer consumes large amounts of data and learns from them according to certain instructions.
After many iterations of learning, the machine becomes able to recognize patterns and relationships in this data. Once the machine spots certain data patterns, structures, or relations on a new data set, it can generate warnings, predictions, and/or make decisions based on prior experience.
Machine learning is highly popular in the following industries:
- Image and speech recognition;
- Natural language processing;
- Predictive analytics;
- Fraud detection;
- Healthcare;
- Finance;
- Manufacturing;
- Robotics.
Most popular ML data analytics trends & examples
Here are some of the latest advances in machine learning technologies and how they are used to improve data analytics in business and IT:
- Deep learning: This method involves neural networks that learn from vast amounts of data before they mature. It is particularly effective for analyzing unstructured data, such as images, audio, and text. Depending on the organization of deep learning results, the ML solution can be used for reproducing or imitating the data it was taught, including creative processes similar to those of the human brain.
- Transfer learning: This ML technique enables ML-based applications to reuse the knowledge learned from one task to amplify performance on another task. The major advantage of the transfer learning method is reducing the amount of data required by neural networks for training. For more accurate results, it’s necessary to involve skilled ML engineers who can curate the process of transfer learning in ML.
- Reinforcement learning: This ML paradigm is based on the natural principle of rewarding desired behaviors and punishing undesired ones. This type of machine learning involves an agent (usually, a human operator) interacting with an environment to learn through trial and error. This practice is being actively used in areas such as robotics, gaming, business operation automation, and transportation & logistics.
- AutoML: This approach covers several techniques and tools that automate machine learning operations, from data collection and preparation to ML model selection, including a very specific ML process called hyperparameter tuning. AutoML can help businesses with limited resources to embrace the power of machine learning more effectively. However, the AutoML environment must be designed and deployed by experienced ML engineers, so it can deliver the best results possible.
- Federated learning: As the term suggests, this is a distributed, collaborative machine learning model that allows multiple devices or applications to cooperate on an ML task without sharing their specific data and violating its privacy. It is particularly efficient in situations where data security is a primal concern, like healthcare, law practices, or advanced financial technologies.
In other words, ML is required to prepare and train the “electronic brain” for augmented, automated decision-making. Machine learning is a necessary prerequisite for the successful functionality of AI systems. The better a machine is trained on big volumes of valid data, the better advice and insights it can give you in the future.
Successful examples of ML projects include two famous products: MidJourney and ChatGPT. If you want to create an ML-based system or application for fin-tech, eLearning, entertainment, logistics, or any other industry, make sure to contact the Forbytes ML team for a consultation.
Is AI Capable of Replacing Human Professionals?
While an ML mimics the process of organic learning, artificial intelligence (AI) is designed to imitate the process of organic thinking. The goal of AI is to teach a computer to replace human reasoning with similar automated processes and let a machine perform certain tasks on its own, without constant human intervention. Most of the AIs are based on neural networks, which are architected after the biological capabilities of the human brain.
Thanks to rapid electronic processes and high-capacity microelectronics, AI solutions can seriously accelerate many tasks traditionally performed by humans, yet not replace professionals completely. In other words, AI for data analytics allows human decision-makers to do more in less time and process tremendous volumes of information, which previously required many hours of manual operation and big numbers of costly specialists.
Here are some of the latest advances in data analytics and AI application examples:
Natural language processing (NLP): talking to robots
With the help of ML and other related techniques, AI can be trained to understand human language, either written or spoken, including emotional tones and hidden meanings. NLP methods empower AI to analyze large arrays of unstructured (user-produced) data automatically, such as social media posts, customer letters, and support tickets. This may include automated answers or other AI-triggered reactions.
For example, the elevation of certain crucial or complicated requests to human operators. NLP and AI can help companies enhance their chatbots and virtual assistants to interpret and answer human messages more organically instead of through hardwired algorithms. The NLP method is widely used in innovative marketing practices for measuring brand reputation, “vox populi” research, and finding out other important customer metrics.
Computer vision: Big Brother is watching you
This is another important trend in AI engineering and development. It enables computers to interpret and investigate visual data in areas such as video surveillance, self-driving cars, facial recognition, and quality control in manufacturing.
Computer vision is also widely used to analyze optical data from the web (like user-uploaded photos) and satellite images to gain insights into a great variety of realms: from geospatial research or ecology situation monitoring to studying enemy military unit disposition or customer interests. Computer vision can also be integrated into modern gaming apps.
Generative adversarial networks (GANs): helpful discrimination
Technically speaking, GAN belongs to the AutoML methods, however, it is AI-driven and used by computers to self-educate. GANs are two opposing neural networks where one is a generator and another is a discriminator. While the first one generates synthetic data objects, the second one tries to distinguish them from real data samples.
In this way, both neural networks compete with each other in a game-like manner. This method is common for Augmented Reality projects and can be used in AR app development for business.
Predictive analytics: AI tells your fortune
Humanity has always been dreaming about an ideal oracle who can flawlessly tell one’s fortune. Finally, AI holds a true chance to become an unbiased and efficient predictor. Thanks to a wide toolset of machine learning algorithms including data fabrics, AI technology is potent to analyze data and make quite precise data-based predictions about future events and outcomes. Predictive analytics can help business executives optimize their business operations, improve sales, customer experience, and mitigate potential organizational risks before they ever emerge.
If you want to develop any of these solutions in data analytics and AI domains, make sure to contact our AI application development engineers. We’ll accurately learn the requirements of your project and provide you with an efficient project conception. Our professional approach to AI system architecture will let you shortly obtain a system design and implementation plan for cost-efficient data analytics, educated business predictions, and more.
Big Data Analytics & Trends
Big data is a specific type of business or technical data that features the following key characteristics:
- Enormous volume: Big data sets are typically huge, with database sizes ranging from terabytes to petabytes, on average.
- Velocity in collection: Big data sets can be generated and captured at a high pace, often in real-time or in nearly real-time modalities, like a high-resolution video being streamed by dozens and hundreds of cameras.
- Variety and inconsistency: Big data sets can include structured, semi-structured, and unstructured data arrays.
- Veracity: Big data sets can be raw, noisy, and contain a lot of errors, artifacts, or uncertainties, which makes it challenging to process and validate.
The data warehouses used to collect big data are called “data lakes.” These huge storages mostly include raw data collected from smartphones, cameras, sensors, medical devices, industrial robots, etc. Here are some of the latest trends in big data analytics applications:
Cloud computing: big data for everyone
Cloud technology enables businesses to store and operate large amounts of data on remote servers in third-party data centers, which is more cost-efficient than keeping corporate infrastructure onsite. Cloud computing is quite an affordable strategy that can be used by many small & medium organizations to access, store, and manage big data.
Thanks to easy accessibility, a wider range of organizations can reserve cloud capacities and make use of real-time data analysis. This trend stimulates the explosive development of SaaS analytical platforms and custom solutions, like SaaS solutions for hotels, medical practices, e-commerce, and more industries or business sectors. If you want to migrate your databases or applications to the cloud, contact our IT engineers for a consultation on bid data and cloud options.
Internet of Things (IoT): how they make big data
IoT is a network of interconnected devices and sensors that can collect and exchange data to coordinate their operations. IoT hardware of higher layers (hubs) synchronizes and collects data from smart cameras, wearables, robotic gear, and other connected devices in real time. These IoT device networks can generate considerable amounts of big data.
Since it’s very large and poorly structured, it’s necessary to send this data to the cloud and apply multiple algorithms to clean, normalize, aggregate, and prepare it for use in analytics. Examples are facial recognition or dynamic GIS navigation for unmanned vehicles.
Sometimes, this process has to be executed very fast, so cloud computing connected to data lakes can be the right solution. Data analytics in IoT, supported by cloud platforms, helps to improve and orchestrate supply chains, autonomous (unmanned) transport routing, self-reliant manufacturing processes, and device user experiences.
Data Visualization: The Future of Data Analytics Born in the Past
Data visualization techniques are not new and have been out there for centuries. However, in the previous epochs, they required well-developed math and graphical skills to be applied.
Nowadays, most data visualizations can be created with a simple click or two. This approach enables organizations to represent and communicate complex information in a comprehensive and engaging way, through pictures, infographics, graphs, charts, and so on. Here are some of the latest techniques in data visualization:
Interactive visualization: just click to get an insight!
This type of data visualization describes software interfaces that enable users to dig into databases, explore records, and interact with data via dashboards. Users can filter, sort, and zoom in on data to gain a better understanding of patterns and trends. Interactive visualization can include numerous types of charts and graphs that can be immediately built at the user’s request.
Any person with at least an intermediate level of education, who can read graphs, can make great gains from using such data analytic interfaces. Interactive visualizations can be an integral part of any popular product or user access portal providing overviews of user activities, like purchase history in e-commerce applications.
Creating efficient interfaces takes serious UI/UX skills — if you need any help with UI design, please consider our user interface development services.
Data storytelling: once upon a time…
Data storytelling is the use of narrative techniques and artistic scenarios to convey insights and tell a story through creative data visualizations. Data storytelling can include rich representations that go far beyond “slide shows” or motion graphics. That could be a short movie or a commercial where you showcase personal stories and amplify your narratives with powerful visual content.
This type of data analytics & visualization works well with specific target audiences: investors, potential consumers of premium goods, politicians, media representatives, and more. Data storytelling helps engagingly highlight important information and persuade stakeholders to take action based on beautifully represented data insights (for example, stimulate them to invest in your new project.)
Augmented reality visualization: breakthrough in eLearning
Augmented reality (AR) visualization uses digital overlays to display virtual data in the context of a real-world environment to boost the organic experience with computer-generated objects. Augmented reality visualization is being used to associate data analytics and representation with real settings. This enables users to envision information in a more immersive and interactive way.
AR can be extremely helpful when it comes to e-learning software development. It can work with special AR devices like smart glasses or usual smartphones and other handheld devices.
Data Governance and Best Practices in Trend Data Analysis
Data governance refers to the policies, best practices, and procedures that organizations must use to manage their data assets and prevent serious violations.
Privacy regulations
There exist numerous laws that you must obey when doing your data analytics and AI/ML research. Just to name a few: CCPA, HIPAA, GDPR, and many more. They are designed to prevent data breaches and eliminate concerns around data privacy.
According to these regulations, you must obey serious constrictions in operations with personally identifiable data. For example, you must anonymize all demographic data before sharing it with, let’s say, your marketing analytics providers until you obtain explicit consent from the owner and follow many more requirements.
Data quality management and stewardship
Data quality management ensures that data is accurate, complete, and consistent. In addition to this, data stewardship is the practice of assigning responsibility for managing data assets to specific executives within an organization. Both practices are required to improve operations with protected or sensitive data and ensure that no data breaches can happen (remember that legal penalties for data security violations can be very painful.)
Conclusion
As you can see, artificial intelligence, machine learning, cloud tech, and data visualization are here, among the current and future trends in data analytics.
Today is the right time to embrace the change and start your IT project involving certain forms of data analytics technologies. However, it’s essential to respect data governance methods and privacy regulations.
If you require immediate assistance with building — contact Forbytes.
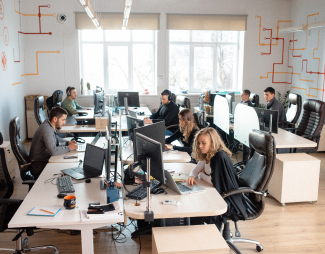
Our Engineers
Can Help
Are you ready to discover all benefits of running a business in the digital era?
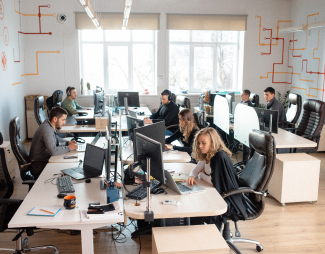
Our Engineers
Can Help
Are you ready to discover all benefits of running a business in the digital era?