The digital age has been anything but black and white. It’s been a time of unprecedented change and rapidly widening insights. We’ve seen big data play a phenomenal role in the ability to understand how consequential a decision is. For example, by combining big data analytics technologies, big shops can now define the customer’s likelihood to buy certain products. That being said, the world has made a massive leap in terms of tech adoption.
Enterprises and governments rely on big data to make better decisions. Big data helps businesses understand customer behavior and market trends, and against that backdrop, companies are competing to create innovative products, services, strategies, and solutions.
But there are a number of problems associated with big data. Companies leveraging the power of big data will need to address them. Let’s discuss the most crucial ones.
Data Quality Problems
1. Data Silos and Poor Data Quality
Data is the backbone of any enterprise. It is an invaluable asset that business leaders can use to make decisions and guide investments across the whole organization. Unfortunately, the ubiquity of data has led to increased data silos and poor data quality. Data can be collected and stored multiple times across different applications and forms, further limiting access and context for employees. Data silos are the unsolved challenges of big data management, causing companies to lose out on opportunities for competitive advantage.
Practical statistical analysis is usually a two-step process. First, there are data cleaning and data preparation steps. Then, the data is analyzed via relevant statistical software. A key is a process-based approach, so data preparation steps and statistical analysis must follow a similar process. When these are not done consistently, data silos and poor data quality can have devastating consequences.
A solution for data silo isn’t just about merging data. It’s about repairing a broken business by connecting information throughout the entire organization. Data gaps are the enemy of successful organizations. Make sure to stop data silos, improve data quality and transparency, and involve everyone who can be helpful in the decision-making process.
Solution: Create a data management system to set policies, procedures, and processes to set the bar for data quality. Make it visible and aligned with business needs and establish strong protection.
2. Outdated Data and Inability to Operationalize Insights
The challenges of conventional systems in big data often lead to even bigger issues. Companies like retailers, banks, and insurance agencies have struggled to adapt to the new marketing landscape. Disparate data sources, lack of standards, and outdated technology make it challenging to understand how brands operate. Big data filled with outdated information can be a management nightmare and make it difficult for vendors to operationalize insights into actionable strategies.
Some researchers lack the tools to find an accurate temporal dimension to the data they work with, while other instruments cannot easily extract data. These two limitations – the inability to mix historical and current data and the inability to analyze the data quickly – have reduced the number of studies conducted.
Solution: Use the agile approach to data management: this includes establishing DataOps and MLOps practices. With their help, you will be able to detect outdated data patterns easily, automate the data management process, and reduce the cycle time of big data analytics.
Working with Data: What Issues You May Face
3. Lack of Proper Understanding of Big Data
Many of the problems associated with big data that companies face today are due to their lack of understanding of how it affects society. Big data is a multi-billion-dollar industry, but many people struggle to understand what big data is and how it creates value. You need to get yourself acquainted with this idea.
A lack of understanding of big data is one of the significant reasons why most organizations haven’t been able to extract value from the data. The term is also poorly understood by many people in marketing and business. Having a clear understanding of the difference between data and big data is the only way companies can harness all the power for their success.
Solution: With a shortage of skilled data professionals, large corporations are investing big sums in the recruitment process. They aim to fill the gaps that exist in their teams. Companies that don’t have that vast resources need to invest their time and money into staff education. For example, they can organize webinars or training programs for their employees.
4. Management of Large Volumes of Big Data
Companies are in the midst of the biggest data storage boom in history. Massive data sets drive ever-larger business insight opportunities. But technology has only begun to enable the scale of data storage necessary for new business analytics. The challenges in data storage and management have been here for a while. A lack of relevant, cost-effective solutions has created a data storage bottleneck in the corporate data centers.
Every single day, the amount of data on the web is growing. In fact, global data creation is projected to grow to more than 180 zettabytes by 2025. This is creating a global, resource-intensive, and ever-evolving problem. Getting the right context on this data is imperative for areas such as healthcare, marketing, and finance, but it is also incredibly challenging.
Data duplication is another big issue. Some large organizations have hundreds if not thousands of databases full of copies of the valuable information that these companies need to operate. While that’s convenient for the companies, it’s also challenging for IT professionals and your business continuity.
Solution: Big data can only be effectively handled when the data is not only collected but also has a clear structure. To improve data-driven decision-making, companies need to break down data and gradually withdraw insights. Trying to operate too big chunks of information never leads to great results.
5. Finding and Fixing Data Quality Issues
Data quality is one of the most critical metrics for any business as it informs decision-making processes and business decisions. And yet, it’s not uncommon for data quality and data quality issues to go unnoticed for quite some time. Companies often use a good data quality tool to handle and fix data errors that could harm their data or their decisions based on certain data points.
Data quality issues exist due to the complexity of today’s semantic web environment. Organizations and businesses struggle with data quality because they work with a flawed and inefficient data model. In addition, young startup companies are generally short on data quality skills and are often unaware of tools available to them to improve the quality of their data.
Data quality is critical for big data systems, as inaccuracies can lead to inconsistencies, non-reproducible findings, and tough-to-grasp analytics. Even a small percentage of incorrect data affects the bottom line and can cause unanticipated consequences.
Solution: To fix any data quality issue in time, use software that automatically identifies typos and duplicates. Also, make sure your team monitors the data you store. The goal is to identify what data to retain, for how long, and in what format.
6. Big Data Integration and Preparation Issues
Data integration and preparation can be complex, time-consuming procedures. Another big data problem that companies encounter when preparing their data for archiving is deciphering between information on different groups of systems inside their business. Because systems are often incompatible, it’s essential to identify what data is stored and where it is stored to extract the correct information without confusion.
As companies drown in data and gain the capability to harness its power, IT professionals must be aware of potential integration and preparation complications. Data scientists have developed new tools to make it easier to prepare data for analysis. There is an increased interest in data preparation solutions as individuals and businesses are looking for more streamlined ways to prepare data for businesses.
Solution: Some companies use data lakes to address this issue. A data lake is a technology that collects and stores data over an extended period of time and delivers it to different departments on-demand. It provides a visual user interface to find data and make use of it quickly.
7. Converting Big Data Into Valuable Insights
The most challenging thing about data is not the data itself but understanding how the data relates to the company’s current and future business opportunities. To use big data to find actionable insights about a business, you need to be able to filter through the data and find patterns or trends that can help you make better decisions.
When working with data, the most important thing you need to consider is the context of the data within the organization. For instance, healthcare organizations aggregate data from a number of disparate sources and use the data to improve patient care. In this case, the big data and analytical tools that will be used for its operation need to be adjusted for monitoring and screening the patient’s experience while being in the hospital.
From science labs to marketing firms, many organizations are grappling with the challenge of turning their enterprise data’s enormous volume and complexity into information that can be turned into insight. Taking data analytics to the next level is not easy. Skilled workers and technologies are in shortage, and the industry is still waiting for shits in this area. Changes must come as soon as possible if we want to solve the challenges in big data analytics and take advantage of this fantastic technology.
Solution: Make sure your big data tool is able to analyze data from social networks and websites. Create a system of important data sources (including external sources) and factors that will allow you to draw the needed insights.
Long-Term Perspective: Challenges to be Ready for
8. Scaling Big Data Systems
There are many challenges associated with scaling, like adding new data sources, building and maintaining infrastructure, developing new solutions, and adding users. There are other challenges that get in the way, like a lack of process, tooling, and standardized procedures. With the need for fast and scalable data systems becoming more crucial, cloud services and open-source software are leading the market. However, those solutions are often too expensive.
Data scientists must deal with a wealth of data from many sources and many data models. Scaling your big data systems or applications can be a significant challenge that might prove tedious or even impossible to conquer. Still, there is a process you can use to help companies overcome the threshold of data that is too much for their current setup.
Solution: Sharding is considered one of the most potent performance-optimization practices in the big data field. Sharding is the act of distributing the workload across multiple servers running identical software. Sharding-based solutions are created to support large amounts of big data storage and operation effectively.
9. Assessing and Selecting Big Data Technologies
Companies and enterprises need access to the tooling and insights necessary to deliver data-driven decisions. But the broad range of tools, data volumes, sources, and platforms makes it difficult to choose the best solution when implementing a big data analytics project. In addition, technology becomes obsolete within a few years, and many of the systems underperform to some degree with the emerging solutions. This danger is amplified in an enterprise setting where the big data technologies are deployed alongside existing legacy systems.
It is difficult to score software products in a fair manner. If there are no agreed-upon minimum criteria, then software utilities can score higher against a general requirement due to a combination of features. First, they need to understand the available tools and how they fit with their goals. This includes thoroughly understanding what it will take to integrate the technology into an organization’s IT environment and culture. Understand the types of data being produced and the events happening in the data center before deciding on a big data technology.
Remember that different types of organizations may fit with different big data technologies. Just like when choosing the tech stack for your software, the type of big data technology must fit your enterprise needs too. It will largely depend on use cases and implementations. If you want to choose the best big data solution, you should take some technology and functionality considerations into account. The most important thing is to consider how your business data is structured and fragmented before contacting a vendor.
Solution: You might consider consulting a specialist before making any decision. Only professionals in the field can help you determine what technologies to use and how to revise and redesign your processes to fully take advantage of them.
Final Word
The foundation of any company today is data. Businesses need to collect, store, manage, and make sense of data from various sources to make the right strategic decisions and grow their business.
The challenges of big data listed above are solvable. However, it might take years and billions of dollars to actually find the best solution. The sheer amount of data created today and the sheer number of ways to harness it is staggering. One thing to be certain about big data: it’s large in scope and poses new opportunities for industries to thrive.
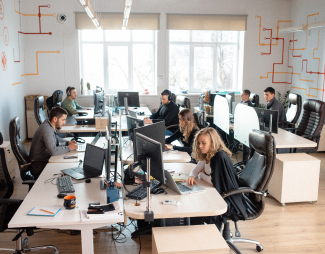
Our Engineers
Can Help
Are you ready to discover all benefits of running a business in the digital era?
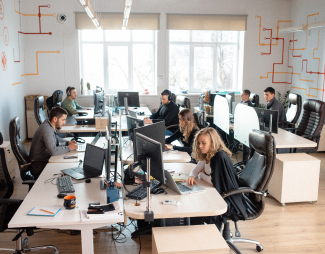
Our Engineers
Can Help
Are you ready to discover all benefits of running a business in the digital era?