Demand volatility is a common challenge that can be difficult to deal with and expensive to manage. However, it can be mitigated in certain ways. AI and ML are the keys to obtaining precise business insights through the analysis of big data sets. And innovative companies stand to gain from this type of demand forecasting method immensely.
What Are Demand Forecasting Methods?
Demand forecasting has become a fascinating data science with ever-increasing business benefits. Various demand forecasting methods are used by companies to understand consumers’ buying behavior. These methods help companies make effective data-based decisions about their market, product, price, and promotion strategy.
Demand forecasting is the process of estimating how much demand for a product or service is likely to occur in a certain period. In order to adjust for seasonal ecommerce trends, planned production changes, or new product launches, most demand models are too slow to account for the real-time changes in customer demand. As a result, companies often see a dramatic shift in their market share that leads to missing opportunities. But imagine if you could have an algorithm that adjusts in real-time to what your customers want.
That’s the power of machine learning, and it’s changing the game for the demand forecasting method. Businesses are making significant investments into machine learning and artificial intelligence to reduce modeling costs, improve accuracy, and deliver a dynamic supply-demand balance.
Machine Learning for Demand Forecasting
If you are a sales leader, accurate data analysis is critical for your work. It’s the starting point for not just making smart, data-driven decisions but also for planning budgets and forecasting performance. Machine learning enables businesses to get ahead of the competition.
For decades, sales leaders have been using data science techniques to help automate B2B sales. Thanks to an improved understanding of how to measure demand, new forecasting software solutions are capable of measuring customers’ intent and using that information to predict which products win and which ones lose. Now, quantifying cognitive insights and using data science to better predict the future of an organization are entering the mainstream.
So, why is ML so important for demand forecasting?
- ML for demand forecasting can help you anticipate changes in system volume, the size of the market, and price points. The process is different from other forecasting methods, so test and compare concepts with real-time data from your own sales and marketing plan.
- ML helps process data, make predictions, and forecast future behaviors. This future of machine learning will create predictions, not just models, with clear results from both human and machine learning, such as new powerful sales tools and decision-making frameworks.
- The newest and most powerful ML is being used to snuff out the black swans and funnel customers to the right place with the lowest wait times. Machine learning models are capable of analyzing a number of characteristics of highly-correlated datasets and produce easy-to-understand forecast tools for demand.
- Marketers need to be aware that demand often doesn’t appear in a way they expected in their campaigns. By using ML, they can leverage past individual and pattern behavior to better understand future demand so as to optimize their marketing efforts.
- Demand forecasting methods are important in any business, but particularly so in the e-commerce industry. How can an e-commerce company know how much inventory to carry on hand, how much to order, or how much to produce? This kind of prediction is central to the effectiveness of any e-commerce strategy.
- Adding ML to your demand generation toolset not only helps marketers better understand demand but it may also make your business more profitable over time. ML technology uses data and patterns to predict future demand with uncanny accuracy, so companies can significantly improve their demand forecast with minimal risk.
Demand forecasting is an essential part of forecasting performance in many industries. This is especially important for industries like logistics, manufacturing, and distribution, where decisions rely on models that forecast demand before the production process. For instance, the traditional model of supply chain management looks as follows:
Meanwhile, demand forecasting based on ML allows manufacturers to ensure they have an adequate product on hand to meet customer needs, thus ensuring quality and timely delivery.
However, in this field, forecasting is difficult as both demand and supply can shift due to changing economic conditions and long-term changes in customer behavior. To make sure that prediction algorithms generate accurate insights, companies need to choose the appropriate methods and solutions.
Retail Demand Planning
Demand forecasting has always been a challenge for retailers, but ML is changing the game. It is designed to predict demand based on historical data, and at this point, retailers are on the leading edge when it comes to using ML to predict demand.
As a result, ML-driven predictive models deliver 50% more accurate forecasts than traditional methods. More notably, an AI/ML-build approach increases profits, which is basically the whole game for retailers. For instance, Tapestry improved margins by adopting an ML-based inventory optimization solution. Using AI/ML suggestions to transfer stock between shops can significantly lower the risk of overstocks or stockouts.
For marketers, ML-based demand forecasting is especially useful in retail because it helps to:
- understand the roles, features, and demographic targets of their target audiences.
- predict audience size, campaign performance as well as competition.
- understand the potential audience and predict their sales.
ML-Driven Demand Forecasting Model
Established forecasting methods have gaps in customer behavior and data, so a new multi-layered approach is needed. Marketers use a variety of methods to improve their market understanding, but accurately forecasting demand is in short supply.
Let us lead you through the three basic steps to creating an accurate ML-based prediction model. The main prerequisite for creating such models is the right set of data.
- Detecting and treating outliers. Outlier treatment is very important for creating sound ML forecasting models. To do this, the most typical approach is to utilize statistical information on data, such as interquartile range and box plots, and reduce outliers according to acquired info. With these methods, most data remain within range, and the impact of high data turbulence is diminished.
- Building the ML model. The output from the outlier detection/treatment data is provided for this step. Research analysis of data is carried out to identify patterns and trends, analyze them, and then figure out which ML model to employ. An autoregressive integrated moving average (ARIMA) model is the most widely used method for stationary data, which follows any trend/pattern through a set of intervals.
- Evaluating the model. Finally, the accuracy of different models needs to be checked. It is necessary to obtain the model that works best by calculating the Mean Absolute Error (MAE) or Root Mean Square Error (RMSE).
In short, the whole process can be reduced to the following steps:
- Develop a demand strategy: set goals, plan horizons, and set a hierarchy.
- Create a demand plan: find out the sources of demand data, create a baseline projection, and construct frequency and prediction procedures.
- Enrich the demand plan: supplement the existing data sources with new parameters.
- Build consensus of demand plan: stimulate forecast consent and consensus processes, including governance.
Data Quality Parameters
Data quality measures are perhaps the most vital parameters in a forecasting model. See, forecasting models can be highly complex and difficult to understand. So, parameters like the maximum level of accuracy of data and estimation intervals are critical.
To make these parameters easy to understand and use, it is necessary to:
- Ensure the data are accurate. In a forecasting model, the data used are typically the data of the past. However, these data may be imprecise. The data could be out of date, something could have been misreported, or there may be errors. The data must be of good quality, or the model will be badly faulty.
- Order your data with parameters that help get the best forecast. These parameters are specific to your forecast requirements and forecasts. For example, it might be worth understanding the differences between new and conversion/revenue forecasts.
- Assure data consistency. Having a data management plan is beneficial for both raw and forecasted data. By ensuring data consistency, you avoid making modeling mistakes that can have unintended consequences for your forecasting model. By ensuring data accuracy, you can reach more accurate forecasts that result in better, more realistic, and more critical decisions.
- Check for data validity and relevance. When it comes to forecasting models with predictive accuracy, data validity, and relevance play a large role in the success. Data accuracy helps identify the relevant data to use or discard. Data validity ensures the intended outcomes are observed with the data set. Data relevance is the degree to which the outcomes are linked to the original goals of the forecasted model.
The following parameters will be used to evaluate the data when creating a forecasting model:
- Sequence;
- Precision;
- Validity;
- Topicality;
- Accessibility;
- Completeness;
- Detailing.
In fact, the data gathered by businesses are frequently not perfect. These data sets usually need to be cleaned, analyzed for voids and anomalies, checked for compliance, and repaired.
Choosing ML-Based Demand Forecasting Software
Many solutions are available to help you handle your demand planning activities. They have different abilities, and you should choose one depending on your current and future needs. Consider the following factors when picking an ML-based solution.
Functionality
The first thing you have to do is to assess if it meets your business requirements. Depending on the industry in which you are operating, you will possibly require certain tactics, strategies, and methods that vary in each separate ML system. You may need:
- short-term or long-term forecasts;
- forecasting the demand for newly-introduced products;
- multilevel plan for diverse areas, media, and product categories;
- assessment of price elasticity;
- multilevel models and similes of “what if” scenarios;
- predicting demand fluctuations, detailed information panels and reports, etc.;
- products cannibalization chart.
Compatibility
It is very important to tie your internal systems (such as ERP or sales management tools) to the demand forecasting system to ensure data exchange, collection of complete historical data, and formation of demand trends. In addition, full integration with your stock control systems will allow you to optimize procurement and avoid over- or under-stock.
Today, it is easy to find a vendor of forecasting solutions that offers ready-made integration with the most widespread ERPs, Excel, and other business means. So, see if your chosen vendor can help you connect to the systems that you already use or may need in the future. Otherwise, you will need to hire IT professionals to build internal integration with your legacy systems. This, of course, means you’ll have to spend more money. Consider various options before making the final decision.
Tech Support and Training
The process of ML implementation is different for every organization and can be a difficult challenge, especially if you’ve never done it before. As a result, IT department teams may face some unique challenges they can’t handle on their own.
Algorithms can learn and improve dynamically without any human intervention, but humans can’t. ML solution vendors need to interact with their clients, understand their problems, and train them as well. Also, since software development changes with time as the industry grows and different programming languages are used, it is vital to have access to tech upgrades in the future.
Data Sources and External Factors
External factors that may have a significant impact on your business vary depending on your industry. We already mentioned the global pandemic, but these factors can also include weather, macroeconomic trends, economic shifts, etc. In this case, it is also necessary to make sure your ML solution vendor employs data sources that are most relevant to your needs.
Generally, it is considered that the more data you have, the more influences will be taken into account and the more precise your predictions will be. But creating such a complex, specialized analytical infrastructure can be pretty expensive. It also requires the involvement of ML engineers, data scientists, and other professionals. Indeed, it will pay off, but you need to pay attention to your investment capabilities.
Anomalies in Demand Forecasting Systems
One of the main challenges that investors, analysts, and entrepreneurs face is trying to develop a sustainable business model. The short-sightedness of many projections and plans is what stands in their way. This is especially true for entrepreneurs starting out in a market that is notoriously fluctuating. The problem is demand forecast systems do not account for unforeseen shifts in demand.
Anomaly is a state of unpredictability, and unpredictability is what makes integrating demand forecasting systems difficult. A good instance of such an anomaly is Covid-19 and the global lockdown as a result of it. The demand forecasting model can’t know that the demand has changed radically. It can only recognize patterns from historical data. For instance, if in 2019 pharmacies had one indicator of demand for medical masks and antivirals, in 2020, it was totally different.
Therefore, companies may need to change their strategy to make accurate predictions:
- Wait a while before collecting product data on new market behaviors, and only then create a model for demand predictions.
- Use a feature development method by counting data such as news, current market conditions, price index, exchange rate, etc.
Despite the fact that there are numerous inefficiencies in ML systems, they are continuously used to forecast demand and help firms operate more efficiently. The graph above illustrates the core competencies necessary for demand forecasting until 2025. If technology evolves according to business demands, the problem of large margins of error will vanish.
Final Word
If a company wants to know what will be happening a year from today, a machine-learning model can produce this data. Here’s what a machine learning algorithm can do for your business: predict demand for your product or service more accurately, shorten sales cycles by understanding buyer motivation, track competitor performance and engagement, and improve competitive advantage.
If you believe in the predictive future, then you need to explore what machine learning can do for you. Contact Forbytes, and we’ll gladly help you!
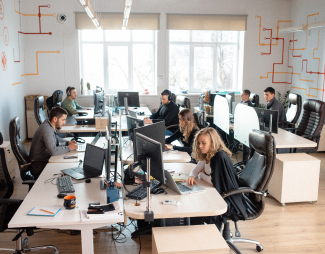
Our Engineers
Can Help
Are you ready to discover all benefits of running a business in the digital era?
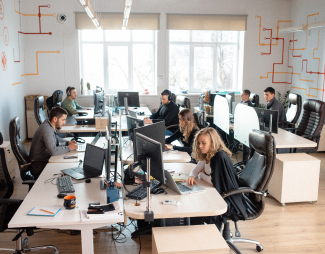
Our Engineers
Can Help
Are you ready to discover all benefits of running a business in the digital era?