The popularity of smart technologies has a big impact on consumer behavior across the globe. By 2023, the e-commerce market in the US is projected to grow to $982,312.2 million, marking an increase of 11.1%. The trend is gaining momentum, and by 2027, US e-commerce will be worth $1,477,360.9 million. More and more merchants move their businesses online every day. They build e-commerce sites, use different e-commerce platforms, sell goods on marketplaces, promote them through social media, etc.
As of 2022, Magento remains one of the most popular ecommerce platforms. Magento 1 powers more than 160,000 active online stores, while Magento 2 is used by more than 100,000 sites. Merchants migrate their stores from Magento 1 to Magento 2 because of the better security, usability, and performance that the latter gives. With the growth of Magento technology, it becomes necessary for online retailers to leverage smart solutions that can facilitate client experience and streamline store management. One of these solutions is an AI-powered product recommendations system Adobe Sensei. This article is an overview of Magento’s product recommendation engine, its benefits, and features. Keep reading to find out if it can be helpful for your business.
What Is a Product Recommendation Tool by Magento?
Sensei is a smart AI and ML technology released by Adobe. With its help, Adobe users can craft and deliver personalized client experiences and reach better engagement with their stores. Earlier, the use of recommendation engines has been a costly decision. It required merchants to hire data scientists or purchase expensive solutions. They also had to integrate these solutions with their Magento store, which required both effort and investment. Starting from 2020, this powerful AI technology has become available for Magento’s merchants. It can help businesses predict client behavior, offer unique experiences using real-time analytics, and optimize back-office processes.
Now, online businesses that use MagentoCommerce can enable automated and smart recommendations on their online stores. Above, you can see the technical specifications of Adobe’s engine. It is available along with other tools and extensions that streamline commerce on Magento. The machine simplifies the interaction with a customer and takes them a step closer to conversion. Differentiated shopping experience provided by AI leads to higher sales and better engagement. Merchants can devote their time to other value-added activities and free their resources for other tasks. Online shoppers greatly benefit from the engine, too. The recommendation of relevant products can ease their shopping journey. They can promptly find the needed products, order product pairs, and get personalized order ideas.
5 Features of Magento’s Product Recommendation Module
Magento’s engine is available at no additional fee for all merchants who use Magento Commerce. If you are wondering about the difference between Magento Open Source and Commerce, check our blog to get a detailed explanation. The engine is available for Magento Commerce 2.3 and later versions. AI-powered product recommendations available on Magento 2 are one more reason to migrate the store from Magento 1 to Magento 2. If you are looking for help with this process with no damage to your data, daily store functioning, and security — Forbytes can help. We have worked with Magento merchants for more than 10 years and have helped them solve various business challenges, from digital transformation to software migration, integration, and building solutions from scratch.
So, what are the features of the Product Recommendation tool by Magento?
- Auto-tagging and catalog synchronization. The AI-powered engine allows for automatic and instant storefront tagging and error-free catalog sync. To add the Sensei technology to your Magento store, you need no coding skills. Catalogs are also automatically updated and synchronized to the cloud service a retailer uses. This reduces the workload on the website server and improves the performance of an online store.
- Differentiated experience. ML technology is leveraged to collect data on client behavior. These data are used by AI to predict consumer trends and optimize the demand-supply balance. This reduces a lot of manual effort for sales and marketing teams.
- 9 recommendation types. Magento’s AI provides product customers with a personalized shopping experience. There are several recommendation types powered by Sensei that online retailers can choose from. Among those, merchants can activate trending product recommendations, most purchased, more like this, recommended for you, etc. The following table shows different types of recommendation widgets and the parts of your store where they can be placed.
Let’s provide a quick overview of each product recommendation type and its meaning. Most viewed products are those that were viewed the most by users within the last 7 days. Most purchased are those that were most frequently bought within the last 7 days. Most added to the cart are the recommendations of the products that were added to the shopping cart page the most within the last 7 days. The trending widget recommends products based on their growing popularity. Recommended for you displays the selling items based on the data analysis of the user’s previous orders and shopping behavior. Viewed X, viewed Y — these are the recommendations that show what items were viewed by the other customers that have viewed particular items before. Viewed X, bought Y — items that were purchased by people who viewed other particular items. Bought X, bought Y widget recommends products that were purchased by the same people who purchased other (particular) items before that.
- Easy recommendation mechanism. To enable different types of product recommendations on your storefront, you will have to go through several steps. Usually, activating the engine takes a few minutes. After the job is done, your users can instantly enjoy this feature.
- Easier storefront management for a merchant. Retailers can create and activate different product recommendation types using the admin panel. There is no need to integrate external AI mechanisms as you have everything needed under one Magento roof.
Main Benefits of the Product Recommendation Tool
There are plenty of ways your business can benefit from having a recommendation system, all of which can be divided into three main categories:
- Increases sales and revenue. Lots of businesses around the world increase their sales and revenue by predicting customer shopping patterns and offering them the right sales item. Amazon, for example, enjoys a 29% increase in annual sales not in small part because of its intricate recommendation system.
- Increases customer engagement. A personalized customer experience makes users more engaged and raises the chances of them coming back and interacting with your store again. Moreover, it raises the chances of converting new visitors into returning customers and improving customer satisfaction.
- Increases efficiency. A recommendation platform powered by machine learning improves the overall efficiency of your business in a variety of ways: from releasing your staff from manual work to making merchandising management more effective. In addition, a recommendation system provides you with accurate reports that you can use to make better decisions and achieve the goals you set for your business.
How eСommerce Personalization Is Done
The workflow of a product recommendation system powered by machine learning (an application of AI) usually consists of 3 steps.
1. Collection
All customer data is collected during the first step. It is assisted by the front end of the e-commerce website, which records all visitor interactions. Two types of data are collected: explicit (comments, ratings, feedback, etc.) and implicit (search history, clicks, order history, etc.).
2. Storage
The text step of eCommerce personalization is storage. All data is saved in permanent storage, which can be accessed by the machine learning platform. There also is additional storage provided for the front end to access related recommendations.
3. Analysis
The machine learning platform analyzes the collected data. Based on the particular needs, there are several methods of analysis:
- Real-time analysis. Data is analyzed “on the go” as it is generated. This method is used to provide quick suggestions and requires tools that can analyze streams of events.
- Batch analysis. The analysis is performed periodically (for example, daily), once enough data has been collected. This method is particularly useful for e-mail campaigns.
- Near-real-time analysis. This method involves gathering and analyzing data quickly (within a few seconds or minutes) and is best used for providing the recommendation of related products during the same online session.
Magento’s Product Recommendation Architecture
If you are a Magento merchant who wants to make use of the recommender engine, you will have to deploy it as a SaaS (Software as a Service). The Magento technology consists of frontend (the storefront) and backend (data services, SaaS export module, admin panel). The template for the product recommendation strategy is a part of the front end. To launch e-commerce product recommendations, the AI technology has to access 2 data types:
- Behavioral data. These are data on client behavior on the e-commerce site. This includes the data on past purchases, product views, and customer cart data. It’s worth mentioning that Adobe Sensei does not collect personal client data.
- Catalog data. These are the data on product name and description, product category, availability, prices, and more.
Once you install and configure the recommender module, Adobe Sensei starts collecting behavioral data from the storefront as well as catalog data. The data are processed, analyzed, and leveraged to create both general and personalized recommendations. Using Magento’s admin panel, merchants can choose between 9 types of automated recommendations to display at the storefront. For each recommendation type you activate, Sensei will collect data and leverage it to make accurate offers.
How to Install the Recommendation Module?
Adobe provides Magento merchants with a user guide on product recommendation installation. To add the product recommendation module, you should go to Magento/product recommendations and install it:
Then, if you want to use the product recommendation module with the page builder, install page builder support by entering the command:
With the help of the page builder and the drag-and-drop functionality, merchants can easily add pages to their stores and customize the features of product pages. They can use the page builder to add recommendation widgets to a web page. If a merchant has a segmented audience, they also can apply different product recommendation types to different client categories by adding dynamic blocks and customizing the widget units.
To regularly update the module and keep it up and running, use composer:
To use the module, you will have to add configurations: an API key that will connect to your storefront and the SaaS environment (check it here).
If you want to get a major update (for example, from version 2 to 3), find the composer.json file of your project. Find the module in this file to edit it and turn it from one version to another. Then save the file.
If needed, you can uninstall the recommendation module anytime.
What about analytics and reporting? Merchants can use the admin panel to see what recommendation widgets are displayed on your storefront. You can manage, add, and remove widgets using an intuitive e-commerce dashboard. Also, in the admin panel of your Magento store, you can see the analytics on each recommendation type. This will help you evaluate the performance of each widget and keep the widgets active that have the biggest impact on your sales.
You get access to the data on:
In Conclusion
Magento gives plenty of opportunities for merchants. Combined with AI, it can also offer a unique and personalized experience for most shoppers with no effort required from retailers. The right product recommendations increase the average order value, enhance the conversion rate, and help clients reach their goals faster. Manual recommendation management is too inefficient and inaccurate. Especially when it comes to growing e-commerce businesses that expand their stock. The use of automated modules can be a game-changer for merchants who want to catch the client’s eye with the right and relevant recommendations.
The AI-powered product recommender system by Magento will do a great job for you and your customers. You don’t have to install external software and go through the taught integration process. Using the Magento admin panel, you will configure and activate this effective AI algorithm. Likely, the emergence of this powerful technology is only the start of AI integration with Magento. We expect more features and opportunities to be available on this e-commerce platform shortly.
Contact our team if you want to keep pace with the latest e-commerce trends and retain customers for a long. We will gladly help you activate the Magento AI module and configure it to serve your needs.
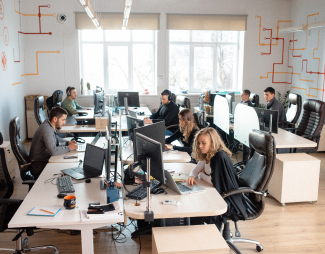
Our Engineers
Can Help
Are you ready to discover all benefits of running a business in the digital era?
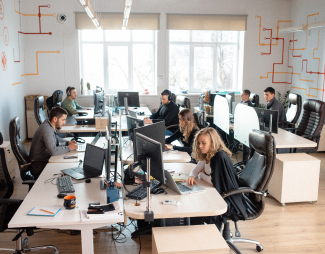
Our Engineers
Can Help
Are you ready to discover all benefits of running a business in the digital era?