Scientists from Johns Hopkins University believe that big data, along with some biomedicine developments, are forming the basis for the Second Revolution in healthcare. They expect such an overture to be as dramatic and comprehensive as the first healthcare revolution with the invention of the microscope. Healthcare will never be the same after big data become a norm in medical records.
What do big data provide to healthcare? In brief, this is about personalization and optimization. Big data processing technologies enable doctors to make the most accurate diagnosis, forecast disease progression, and predict risks for every patient to mitigate negative consequences in time.
Big data allow for recognizing patterns and detecting symptoms based on the massive amount of information collected across the healthcare sector — this is how precise medical decisions occur. However, healthcare providers have to obtain corresponding technical infrastructures to let big data bring the expected value. Hence, the great promise goes hand in hand with particular challenges when big data get into healthcare. We hope the given blogpost will help sort out the benefits and challenges of big data in this field.
What Big Data Mean in General
Even though entirely different interpretations of big data are unavailable, some specific nuances of the definition are worth mentioning. McKinsey, for example, defines big data as datasets with particular characteristics of both the size and structure that make conventional data-processing software unable to collect, store, analyze, and utilize the data.
Gartner, in turn, proposes considering big data as the information assets seen through the lenses of the so-called 3V: velocity, variety, and volume. Such assets require innovative approaches to their processing and analysis.
All experts agree that meaningful insights can be derived from big data only when it is well-structured after specific processing. In such a context, the 4th V can be added: veracity.
A healthcare-specific definition of big data is also available. It has been proposed by the Health Directorate of the European Commission: a large volume of diverse biological, clinical, and lifestyle information collected from people about their health status.
But the first mention of big data can be found in the “Nature” magazine from 2008 that defines any data flow bigger than 150 Mb/day as big data.
How to Use Big Data in Healthcare
The first case of processing big medical data is probably related to mapping the human genome. The process started in 2000, and a decade has to pass before about 3 billion symbols of humans’ primary “genetic document” have been deciphered. Today, the same can be done in less than an hour.
Many experts suppose that big data can and even must cover the following activities within the healthcare sector:
1. Personalization of medical care
This is about personal health records that can be transformed into big data if everything related to the patient’s health status is digitized and shared with relevant medical organizations. If health-related data are collected from day one, many diseases can be predicted at a very early stage. Diabetes, for example, becomes easily treatable if detected in childhood and countered by a timely prescribed diet.
2. Improving diagnostics.
The larger the number of thoroughly recorded medical cases of a specific disease, the better the diagnostics of the disease: two heads better than one, as they say. The Oncospace smart database (Johns Hopkins University, the USA), for example, contains tons of 3D images of cancerous tumors along with anamnesis of related patients. The project has significantly improved the effect of radiotherapy prescribed on the grounds of diagnostics made with the help of the database.
3. Preventing epidemics.
Consolidated databases that cover the population in mass provide the optimization of knowledge about public health. The information collected and processed by big data analytic systems can help epidemiologists understand whether another wave of a virus is likely to come.
4. Tackling fraud in health insurance
Healthcare coverage has always been a matter of manipulation practiced by various fraudsters and cheaters. Unlike pre-computer times, the current digital era equips fraudsters with numerous means of falsifying electronic health insurance documents. Big data analytics help detect fraudsters via multiple digital identifiers that can be automatically applied to health insurance databases.
5. Monitoring medical IoT devices
The Internet of Things keeps covering clinics and hospitals with smart devices that significantly improve patient care. At the same time, IoT devices generate terabytes of unstructured data that have to be somehow processed to be useful for doctors and hospital staff. Such raw data can contribute to the insightful monitoring process when corresponding big data analytic systems work in conjunction with IoT networks.
The University of California, for example, has implemented real-time tracking of the patients’ status based on various health parameters such as heart rate, blood pressure, body temperature, and the like that are gathered by IoT devices and processed by big data algorithms.
6. Enhancing medical research
Healthcare is a science-intensive sector that can succeed only when research data are accessible to the maximum number of medical professionals. Clinics, universities, laboratories, and other healthcare institutions worldwide continuously share their scientific accomplishments via the Internet. Huge volumes of information gathered from various pharmaceutical and clinical experiments fill out special databases that may turn into a mess unless being structured by big data processing algorithms.
7. Boosting telemedicine
In the light of the recent recurrent lockdowns, telemedicine applications are gaining significant momentum. However, the sheer scope of distant healthcare has to encompass some other approaches than just online consultations with doctors. The trivial reason is an insufficient number of doctors for the entire population.
Robotized telemedicine systems such as chatbots, for instance, can significantly facilitate self-service healthcare practices. They can be backed by well-organized public databases enriched by the clinic experience of numerous doctors, hospitals, and pharmaceutical experts. Medicine-related big data seem to be the fuel with which self-service healthcare can run.
The use cases above can hardly limit the approachable methods of utilizing big data in healthcare. More and more spheres of life become data-driven due to irreversible digitization, and healthcare is no exception. The variety of useful big data will keep expanding across the healthcare sector. Today, the following sorts of information can be attributed to big data in healthcare:
Factors and Trends for Using Big Data in Healthcare
In the Top Health Issues report, PwC indicates that 91% of respondents have already used virtual video care at least once. But telemedicine is not the only trend that reflects how healthcare is moving to the future. PwC considers the following factors critical for making the healthcare system simpler while achieving active progress in the sector:
- Adoption of Artificial Intelligence (AI)
- Development of instructions for using AI in healthcare
- Creation of comfortable conditions for patients
- Transformation of clinical trials
- Implementation of technologies for distant care
- Enhancements in cybersecurity
- Using big data on social factors.
According to the report, some ambivalent and even negative factors impact the healthcare sector. Decreased confidence in healthcare technologies and organizations is one of them. People begin demanding more information about treatment and the criteria for choosing a particular treatment. More information implies big data, and it seems big data can never be too big in such a context.
Another alarming trend is aging. The aging population is becoming more demanding of the local healthcare infrastructures and social institutions. The UN predicts more than 1.5 billion people older than 65 to appear globally by 2050. Just to record, identify, and monitor such a group, a huge set of big data has to be absorbed by the healthcare sector.
Yet another impactful factor is the growth of user activity on the Internet. Modern people cultivate a specific behavioral pattern when complaining via social media supersedes visiting doctors. It is becoming common when potential patients seek advice from social-media communities instead of consulting with healthcare specialists.
Counterfeiting such behavior seems to make no sense for any healthcare institution. It is better to follow a well-known principle “if you can’t beat them, join them” when establishing big-data-driven virtual entities to introduce medical knowledge to Internet users.
Besides, those complaints can be collected and analyzed with big data processing algorithms to identify symptomatic cases. Healthcare specialists can create massive prevention campaigns to raise awareness among transnational Internet communities, therefore.
Personal health records are also worth mentioning while realizing how to use big data in healthcare. Presently, health records are disparate and, often, isolated datasets rather than a consolidated, easily shareable database. If it were otherwise, the already existing methods of big data analytics would eliminate the problem of poorly structured medical data.
Big data processing technologies can determine therapies more precisely and identify the side effects of drugs more reliably. Who knows, maybe if big data analytics were implemented in the production, testing, and prescribing of pain relievers in the USA a decade ago, opioid addiction would never reach such outrageous proportions nowadays.
Machine Learning in Healthcare
A separate trend to discuss is the use of machine learning potential in healthcare. People tend to associate ML with voice assistants and complex applications aimed to facilitate ecommerce. But the latest progress made in the sphere of ML and AI also allows healthcare organizations to drive the second revolution in the market. They start introducing new solutions to the most painful patient problems and care challenges. Let’s discuss how ML helps the healthcare sector to turn untapped patient data into valuable and action-driven insights and analyze a few machine learning use cases.
Putting an end to stacks of useless documents
At least once, we all saw the situation when a medical worker looks for the needed patient document by checking the endless (as they seem) stacks of documents on the shelf. Time passes, and now, we rarely come across such situations — all these stacks of files moved to an online mode, forming the electronic health records (EHR).
Yes, by digitizing healthcare, organizations freed a lot of space and saved a tone of employee time. But even now, online healthcare archives are kept for the sake of keeping and bring little value to the treatment process and care delivery. With integrated machine learning technologies, the situation can change.
The most frequent example of how data is used in healthcare is when medical workers study patient records before operation. Information on patient history makes up only 20% of all healthcare data. As a rule, this data is structured and organized in an effective way so that healthcare providers can quickly access it. But what about the rest 80%? The rest of the healthcare data stays untouched and useless because it is not structured and systematized. Usually, it is stored in the free form of text descriptions and notes.
The use of machine learning in medicine changes this industry. The technology allows for effortless insight generation from the analysis of unstructured data. This facilitates the process of providing care and treatment and gives medical workers useful information on patient family history, health details that can affect recovery, the peculiarities of mental state, and so on. The ML-processed data enables healthcare providers to make informed and timely decisions on the best treatment strategy and minimize risks while developing operation programs.
Bridging the gap between medical and social
There are many other ways to use machine learning for healthcare. One of such ways is to provide healthcare with social context with the help of ML. As studies demonstrate, social and economic factors like income or education have a great influence on patient health, their capability to recover, and their readiness to follow medical guidance. This is why medical workers have to consider socioeconomic context while building a personalized recovery strategy.
Deep learning in healthcare allows for the combination of social context and medical cases. ML-powered analysis of unstructured data from various databases enables healthcare providers to learn more about patient context, their habits, and their social status. This may be used for adjusting traditional healthcare approaches to particular needs. The more effective approach, the better treatment results. Healthcare organizations can prevent chronic diseases, eliminate risks, and elaborate on a more effective psychological base for physician-patient interaction.
Improving patient outcomes
One of the most important machine learning uses in healthcare is improving the treatment results. Healthcare machine learning is an example of the value-based approach to providing patient-oriented services. It helps to speed up the treatment and recovery processes, get more positive outcomes, and reduce treatment costs.
As you see, machine learning healthcare is not about creating charts and data analytics on patient outcomes. This is about using these charts and diagrams for practical purposes. ML helps to detect documentation gaps, automate filling in medical forms, and identify hidden risks. Healthcare providers do not have to do lots of paperwork and can focus on the tasks directly liked to patient treatment.
Benefits of Big Data in Healthcare
Optimization of processes is one of the primary qualities of big data processing technologies. Such a quality contributes to the following healthcare fields, inter alia:
- The ability to control one’s own health. Some sort of comparative medicine should appear from professionally processed datasets of various health records collected across the global healthcare sector. Patients can compare their health status with symptomatic cases represented by health-related big data analytics. Moreover, big data algorithms can automate the process while extracting the most relevant symptoms and diagnoses from a vast array of similar cases.
- Simplification of the decision-making process when doctors have to make a diagnosis based on the computer analysis of the patient’s anamnesis. This is when the human experience of healthcare specialists is augmented by the capabilities of machine diagnostics.
- Transformation of traditional healthcare practices into innovative methods of capturing institutional memory and professional expertise. Big data allow doctors to keep and develop self-improving therapies when collective experience enables healthcare providers to achieve sustainable progress in treating various complex diseases.
The vast majority of medical professionals recognize the specific advantages of big data in healthcare. Various surveys among doctors show the primary positive outcomes of using big data analytics in healthcare:
- Time-saving workflows. Big data analytics eliminates manual work in many information-processing routines to free healthcare staff for various professional activities other than working at the computer. Besides, big data algorithms appear more precise and efficient in data processing than error-prone human operators;
- More accurate diagnostics. The more the observed use cases, the cleaner the symptomatic patterns. Any precise diagnosis relies heavily on two interdependent factors: the doctor’s qualification and the amount of data collected about a particular case. Moreover, the first factor remains passive if a lack of relevant information occurs;
- More efficient therapies. Progressive treatment methods need to be shared as widely as possible to contribute to clinical practice. Even a single use case matters: a particular laboratory finding or a report from an ambulance crew can potentially save someone’s life. Healthcare information systems based on big data collected from all over the world help doctors choose the most relevant therapy for each particular patient;
- Reducing the risks of disease. Analyzing pandemic patterns is impossible without big data. The same relates to once-common and chronic diseases such as diabetes, cardiovascular disease, seasonal colds, flu, etc.
- Tracking health-related social factors. Indirectly but effectively, big data provide insights into some social factors that impact people’s health: the nutritional quality (either a propensity for obesity or signs of malnutrition), drug regulation and control (availability of opioid analgesics in the free pharmacy market), local hygiene and preventive measures, climate change & weather conditions, etc.
Challenges of Big Data in Healthcare
Even though healthcare is becoming one of the most data-driven sectors, big data processing technologies have to fight their way to clinics and hospitals. Some specific reasons for it include:
1. Lack of big-data-driven healthcare software.
Although big data technologies keep developing rapidly in general, a specific shortage of medical big data software systems is still observed. Quite rare specialists are needed to create, distribute, implement, and operate such systems. The medical big data analyst remains an exotic specialty for both software-centric educational institutions and medical universities.
To create meaningful big data solutions for healthcare organizations, software development companies need to combine specific healthcare expertise with big data programming skills. Medical science and computer programming stand pretty far from each other in terms of their knowledge bases to be combined with ease.
Hence, big-data-driven healthcare software systems have to appear as just custom-made products created upon request of particular healthcare providers that are ready to share their medical expertise with software developers.
2. Complexities of big data healthcare systems’ implementation
Even a small clinic is quite a complex entity in terms of technical and organizational issues. Implementation of any new IT system is never easy for clinic staff. It entails a whole series of extra expenses regarding necessary hardware and compulsory staff training.
Besides, local regulations and specific industry standards impose particular requirements on software compliance. Moreover, any IT system needs to be correctly maintained and continuously supported to keep the error-free operation in such a sensitive field as human health.
Thus, in certain circumstances, the variety of constraints and difficulties can neutralize the expected positive effect of a big data system’s implementation in a healthcare organization.
3. Lack of well-trained big data specialists
Big data are still an infant technology to have a sufficient number of specialists in any sector. Medical staff capable of doing big data analytics remains rare and expensive. Software vendors organize training courses for their healthcare customers, of course.
At the same time, some basic user techniques instead of a deep understanding of big data algorithmic can be provided by such courses only. Full-fledged big data analysts can appear among doctors and nurses only if medical universities start graduating healthcare-centric big data engineers.
4. Data privacy and cybersecurity
Personal health data are one of the most sensitive types of information ever. Numerous methods of influencing the healthcare system are based on manipulations of private patients’ data. Disclosing some details about heart disease, for example, can result in an increase in insurance contributions due to the risk of a heart attack.
Cybersecurity threats appear when private health records leave isolated on-premise databases for big data repositories. That’s why not every healthcare provider agrees to expose the patients’ personal information to big data analytics, whichever good intentions lie behind it. Big data privacy has to become a standalone topic for big data software vendors working in the healthcare industry.
Conclusion
To address the question “Why are big data important in healthcare?” it is necessary to grasp the realm of healthcare-related datasets: personal health records, laboratory findings, epidemiology stats, etc. Besides, it is worth understanding the scope of data-generating sources: in-hospital IoT devices, ambulance crews, medical universities, pharmacy labs, and the like.
A holistic picture of moving datasets across the industry can show how to use big data in healthcare. For example, personalization of treatment, optimization of clinic workflows, prediction of epidemic waves, and modernization of health records’ processing constitute a few of many possible ways of utilizing big data in healthcare.
Achieving more accurate diagnostics and providing more efficient therapies are the most valuable benefits of big data in healthcare, inter alia. Yet, at the same time, big data adoption brings specific challenges to healthcare providers: a lack of skilled big-data-centric medical staff and extra expenses associated with big data systems’ implementation seem to be the toughest.
Contact us today if you need comprehensive support and professional assistance in developing a pro-big-data agenda for your healthcare organization.
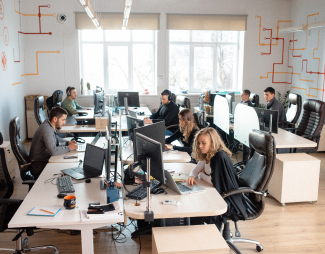
Our Engineers
Can Help
Are you ready to discover all benefits of running a business in the digital era?
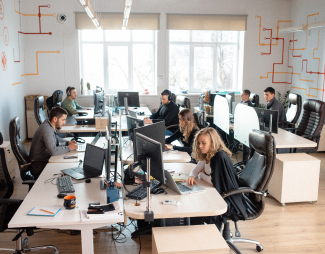
Our Engineers
Can Help
Are you ready to discover all benefits of running a business in the digital era?