Machine learning (ML) has revolutionized industries by encouraging businesses to learn from data and make smart choices. But building ML models can be tough and time-consuming. You need to know about feature engineering, hyperparameter tuning, algorithm selection, and data preprocessing,
That’s where automated machine learning (AutoML) comes in. It makes the whole process easier and encourages using advanced predictive models.
According to AI Multiple Research, 61% of businesses report that they’ve implemented AutoML software or are in the process of implementing it. 25% of companies report that they’re planning to adopt AutoML within the next year. So, these figures prove that automated machine learning is a must-have solution for those who use AI and ML in their business.
So, what is AutoML, what are its major tools and applications, and how to make the most of its advantages for your business? We’ll cover that further down, so keep reading.
What Is AutoML?
Automated machine learning, also referred to as automated ML or AutoML is an emerging technology to automate machine learning tasks, accelerate the model-building process, help data scientists focus on higher value-added duties, and improve the accuracy of ML models. AutoML is trying to automate parts of the data science workflow and contribute to data-driven decision-making.
Automated machine learning is an automated practice of selecting the model algorithm, hyperparameter optimization, modeling by iterations, and model evaluation. This technology doesn’t aim to substitute data scientists but rather frees them from repetitive tasks.
AutoMl is in growing demand because of its features:
- Usability: providing machine learning as a tool to non-machine learning experts;
- Productivity: increasing the productivity of machine learning engineers;
- Performance: finding better machine learning models.
With the emergence of automated machine learning, a new era of R&D and business app development has started. AutoML is about generating solutions without compromising accuracy, making machine learning more accessible, reducing human expertise, and improving model performance in general.
The major advantages of automated machine learning are the following:
- Democratization: it makes ML features accessible to non-experts;
- Error reduction: it prevents possible faults caused by human intervention;
- Adding to efficiency: ML automates running repetitive tasks;
- Optimization: ML tunes hyperparameters;
- Management: managing the model’s future utilization, and more.
AutoML Market Review
According to the latest research, in 2023, the global AutoML market was approximately $1 billion, and it is projected that it will reach $6,4 billion by the end of 2028 with an annual growth rate of 44,6%. If we review this data, it becomes clear that the influence of AutoML will continue to grow and bring more business opportunities.
Problems in AutoML and How to Avoid Them
AutoML does have considerable success in implementing AI advancements. But still, the AutoML implementation process has room for improvement. The question of interplay between data, models, and humans arises.
Firstly, AutoML engineers need help processing unstructured and semi-structured data. The next point is that the modern AutoML framework optimization goals are not constant. There is only one way for effective judgment after the final results are presented.
Furthermore, it’s difficult to implement machine learning models and obtain trusted outcomes as the conditions are changing at high speed. The AutoML applications that are currently offered by the market can only run one machine-learning model program. For example, PyTorch.
Another challenge that should be mentioned is making machine learning models explainable. Partly, it’s even a question of personal judgment. The solution found may not meet the final users’ expectations. Organizations have to work on developing standards related to understandable, consistent machine learning.
And lastly, organizations are experiencing a lack of regulation, standards, and law support when it comes to the privacy and security of machine learning models. Modern technical solutions should be applied to different scenarios.
At Forbytes, we can assist you in handling all these hurdles. Ready to take your business to the next level with AutoML? Let us be your guide. Contact us today to see how we can boost your operations with automated machine learning.
AutoML Processes
Machine learning model selection and automation of the process of hyperparameter optimization, also known as tuning, are AutoML’s most valuable features. This requires the use of various techniques.
Deep Learning and Neural Networks
One type of machine learning is based on the idea that human neurons can respond to triggers and interact with other neurons by sending them signals. This entity of millions of nodes is called a neural network. Nodes can deal with complex problems by splitting them into smaller tasks.
For example, the neural network that is in charge of recognizing dogs might have a layer of nodes determining whether the object is furry. Another layer may look for tails or legs or color patterns. This complicated system develops automatically through constant training with thousands of examples.
Neural networks are good in environments that are:
- highly complex;
- constantly changing.
Machine learning is a fundamental change in computing. We have passed the times when the enormous amount of data collected from different sources could be processed manually. Now, it seems almost impossible and fully ineffective. Unlike traditional software programs, neural networks are scalable: new layers are added without increasing complexity.
The basis of AutoML is a Neural Architecture Search (NAS) set of algorithms applied to neural networks and deep learning. The NAS set of algorithms is given the input set of data and selects the most relevant architecture and hyperparameters. The model is tuned automatically, and these algorithms can essentially replace ML developers.
Meta-Learning
Meta-learning, or the so-called learning to learn, is the ability of various machine learning approaches to work on different types of datasets. It results in learning from the outputs, being more effective, and conducting new tasks much faster. Machine learning models learn from historical data.
AutoML Applications
AutoML consolidates best AI practices to make data science progressively accessible and simultaneously reduce time spent on generating value. There are many tasks at which machine learning is far better than human beings. Each industry is utilizing machine learning in different ways to take advantage of this cutting-edge technology. So what are the most innovative AutoML applications?
Fraud detection is one of the most fundamental applications of machine learning. The future of retail cannot exist without online shopping. With the growth of the eCommerce industry and the increased number of people using credit cards as a payment method, credit card fraud is becoming the most common type of identity theft.
Another application of AutoML is translation. The most known application of ML in automated translation is Google’s GNMT (Google Neural Machine Translation). Fluency and accuracy are reached by utilizing Neural Language Processing (POS Tagging, Named Entity Recognition, and Chunking).
AI plays a great role in the healthcare industry and medical diagnosis management in particular. Whether it goes about critical medical parameters examination, disease progression forecast based on the information extracted, treatment planning, or support, ML holds the key to effective automation of all regular, manual, and tedious workloads.
Machine learning methodologies are additionally utilized to de-risk and accelerate clinical trials.
If you’ve ever used the Uber taxi app, it means that you’ve been utilizing machine learning as well. Uber’s customized application automatically detects a client’s location and offers a destination spot based on his/her previous experience (ML calculation based on Historic Trip Data).
Speaking about transportation, Tesla as a pioneer of self-driving (little or no human involvement) cars is also worth mentioning. Its current AI is powered by hardware producer NVIDIA, which depends on the Unsupervised Learning Algorithm.
Comparison of AutoML Tools
Automated Machine Learning has a long development way, with a lot of great AutoML frameworks only emerging. These tools are designed to make using machine learning models simple and enable data scientists and ML engineers to build scalable machine learning models. Generally, AutoML tools must be able to build models with a wide range of algorithms (decision trees, neural nets, etc.) and provide a refined machine-learning model to the end-user.
It should be mentioned that there is an obvious difference between open-source and enterprise solutions for AutoML. Open-source solutions can only automate algorithm selection and hyperparameter tuning, whereas enterprise solutions can do way more: data preparation and ingestion, column type detection, column intent detection, featurization, meta-learning, transfer-learning, model selection, hyperparameter optimization, pipeline selection, and so on. In addition, the results achieved using open-source solutions are quite worse than those of enterprise solutions.
Machine learning companies are investing in the research and development of ML to bring AI closer to consumers. Let’s briefly describe the use and performance of several of the most common AutoML tools available in the market (in random order).
PyTorch
PyTorch is an ML library, computing framework, and scripting language. PyTorch can be used on cloud platforms It utilizes Autograd Module to build neural networks. It helps in creating computational graphs and is easy to use because of the hybrid front end.
Auto-sklearn
The creators of auto-sklearn are the researchers at the University of Freiburg. Auto-sklearn is an open-source Python package. Its development is done on Github. It was built based on the scikit-learn machine learning library.
The two main features of this toolkit are the automatic search for an appropriate learning algorithm for a new ML dataset and hyperparameter optimization. This tool offers a non-standard supervised machine learning, splitting preprocessing into data preprocessing and feature preprocessing. Auto-sklearn 2.0 is already available. More about technology: NeurIPS 2015.
MLBox
MLBox is one of the top AutoML software and Python libraries with multiple useful features. It’s a framework that solves the tasks of data preparation, model selection, and hyperparameter search.
Amazon Lex
Amazon Lex is powered by the same deep learning technology as Alexa. It’s a fully managed AI service. Its goals are to create interfaces for various communication applications for better conversations.
Amazon Lex solves the problem of speech recognition and language understanding. It helps build virtual contact center agents and IVR, automate informational responses, improve productivity with application bots, and design chatbots. Its key features include natural conversations, builder productivity, and AWS service integrations.
H₂O AutoML
H₂O AutoML was designed as an answer to the demand for ML developers. H₂O AutoML software supports traditional ML models and neural networks. Its main application area is the automation of the ML workflow. Models are supposed to be automatically trained and tuned within a time limit indicated by users.
AutoKeras
Another tool that is worth mentioning is AutoKeras. It was developed by DATA Lab at Texas A&M University. This is an open-source technology that uses the deep learning library Keras.
AutoKeras utilizes the most recent version of Neural Architecture Search, ENAS, but keeps network functionality while introducing changes to the project architecture along with Bayesian optimization. This approach leads to a more adequate neural network search. Applying AutoKeras algorithms requires minimal ML expertise.
Data Robot
Data Robot is an AutoML tool that deals with predictive analytics. It assists data science experts in building and integrating correct predictive models and coordinates time frames in accordance with other software.
Data Robot AutoML owns the constantly-increasing library of recently developed algorithms and provides access to the prototypes for dataset preparation and feature selection.
BigML
The first version of BigML AutoML helps automate the complete machine learning pipeline, not only the model selection. In addition, its utilization is quite simple. BigML’s AutoML performs three main operations: feature generation, feature selection, and model selection.
BigML facilitates unlimited predictive applications across industries, including aerospace, automotive, energy, entertainment, financial services, food, healthcare, IoT, pharmaceutical, transportation, telecommunications, and more.
Google Cloud AutoML
Google developed the Google Cloud AutoML tool using an approach called reinforcement learning. Here, AutoML behaves like a controller, which further develops the child ML model.
Google’s platform is a part of Vertex AI. It has an automated ability to train models on structured data: visual (image and video) and textual. Besides, Google’s AutoML offers interesting advanced configuration options that can be used to potentially simplify existing ML workflows.
Auto-WEKA
Auto-WEKA (Waikato Environment for Knowledge Analysis) was released in 2013 in New Zealand. The second version of the technology, Auto-WEKA 2.0, was released in 2017. The most common application case is tabular data (a table with rows and columns).
Auto-WEKA combines both selecting algorithms and optimizing hyperparameters. The model selection feature is customized. Application maximizes general performance and offers a good indicator of productivity.
Summing It Up
The future doesn’t invent itself — business leaders are those who make it. The global ML market is booming, with more and more businesses adopting this technology in their daily operations. No wonder, ML is regarded as one of the major forces driving innovation today.
At Forbytes, we tackle our client’s business challenges with intelligent ML solutions. By incorporating machine learning, we empower companies with insights into strategic directions for growth and risk mitigation. If you’re eager to develop ML software but uncertain where to begin, we’re here to provide expert guidance.
Beyond integration services, we offer advisory support to assess your operations, processes, and challenges comprehensively. Furthermore, we aid in data analysis and engineering, ensuring that your data propels you toward success.
If you seek a tech partner to integrate machine learning into your business, consider Forbytes. Let us show how to use machine learning, mostly AutoML with benefits for your company.
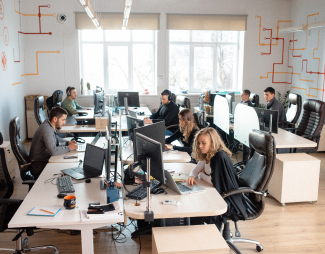
Our Engineers
Can Help
Are you ready to discover all benefits of running a business in the digital era?
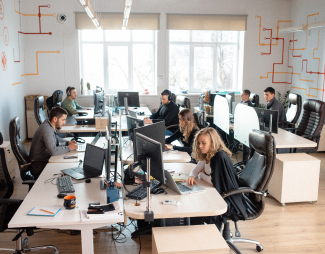
Our Engineers
Can Help
Are you ready to discover all benefits of running a business in the digital era?